[ad_1]
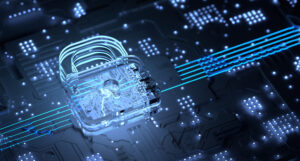
(JLStock/Shutterstock)
Whereas enterprises perceive the necessity to innovate to remain aggressive, they’re additionally cautious about defending their information. Enterprises typically grapple with balancing innovation and safety in relation to extracting worth from their information utilizing generative AI.
Current methods to operationalize the info are both too dangerous or insufficient. Consequently, most organizations are pressured to be cautious and prioritize safety, leading to stalled AI initiatives.
Opaque Methods, a safety information analytics startup, provides an answer to beat these challenges and unlock the complete worth of organizations’ information. The corporate has unveiled its new Confidential AI platform, designed to speed up AI workloads into manufacturing.
The brand new platform was introduced on the 2024 Confidential Computing Summit in San Francisco, CA. One of many key capabilities of the brand new platform is that it allows enterprises to run a variety of AI workloads, akin to SWL analytics and AI inference, on encrypted information with no need any reengineering. It additionally helps machine studying pipelines and common languages and frameworks for AI, together with Spark and Python.
The Confidential AI platform was developed on the Berkeley RISELab, a world-renowned lab recognized for growing applied sciences akin to Apache Spark and Databricks. It was at this lab the place the breakthrough MC2 (Multiparty Collaboration and Competitors) platform was created, incubated, and open-sourced. In 2021, this platform served as the muse to construct the Opaque platform.
Constructing on Opaque’s current providers that facilitate safe collaboration with cryptographic verification of privateness, the brand new platform permits organizations to unlock enterprise insights securely and effectively from delicate information that wasn’t totally utilized earlier than.
Final yr, Opaque introduced key improvements to the platform together with broader assist for confidential AI use instances and new safeguards for ML and AI fashions from publicity to unauthorized events.
“Opaque provides a breakthrough for organizations fighting the strain between innovation and safety. By embedding privateness and safety into each step of the ML pipeline, we allow enterprises to speed up AI adoption confidently,” stated Chester Leung, co-founder and Head of Platform Structure at Opaque.
“Our confidential AI platform uniquely allows the processing of encrypted information with no noticeable efficiency hit at cloud scale. With Opaque securing complete information workloads, corporations can unlock new enterprise alternatives and handle dangers successfully, all whereas sustaining absolute management and privateness of their information.”
Use instances for the brand new platform span throughout numerous industries. Within the high-tech sector, Confidential AI can be utilized to safe information pipelines for analytics and ML workloads and allow dynamic mannequin coaching on encrypted information.
Customers within the manufacturing sector can use the platform as a confidential management aircraft to implement information governance guidelines. Monetary providers can even profit from the platform by safe information sharing and collaboration throughout enterprise models.
Human assets professionals can harness the facility of the platform to securely share and analyze worker information throughout a number of information silos and guarantee enterprise compliance with information privateness laws.
With the launch of the brand new platform, enterprises might lastly have an answer to remove the tradeoff between innovation and safety. As extra organizations use the brand new platform, we can have a greater understanding of the efficiency of Confidential AI when it comes to integration with current ecosystems and scalability.
Associated Objects
VAST Information Companions with Superna to Improve Cybersecurity for AI Workloads
Cisco Broadcasts Nexus HyperFabric AI Clusters with NVIDIA for Enhanced AI Workloads
Immuta’s New Integration with Databricks Offers Safety at Scale for Information and AI Workloads
[ad_2]