[ad_1]
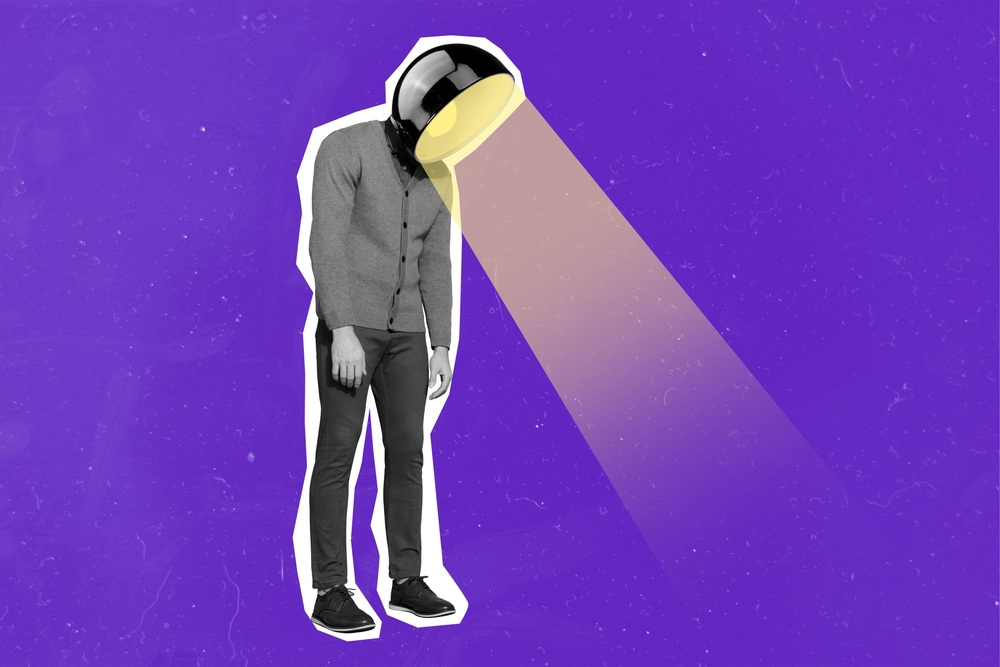
(Roman-Samborskyi/Shutterstock)
Doesn’t it appear to be there’s a brand new machine studying mannequin launched each week? That’s in all probability as a result of there’s.
From Sora to LLaMA-3 and Claude 2, fashions at this time are available in all sizes and shapes—open supply, off the shelf—with various efficiency charges, price implications, and charge limits. Every supplier makes large guarantees to revolutionize the business, and your online business particularly.
However the actuality is that mannequin fatigue is setting in. Selecting a mannequin at this time is like strolling down the cereal aisle on the grocery retailer. We’re spoiled for selection, and selection is nice. However in contrast to cereal, you’ll be able to’t simply throw a mannequin away if you happen to don’t prefer it. Investing in a know-how takes assets and experimentation, and any errors may lead to vital price to your online business.
This prompts a central query: how does any enterprise know the way a mannequin goes to carry out? Even when normal benchmarks are excessive, how do they comprehend it’s proper for his or her enterprise? Nicely, they don’t. And herein lies the issue.
The Exhaustion of Having Limitless Selections
We’re overwhelmed by the sheer variety of choices and what goes into selecting the best mannequin for the job. This takes onerous work. A enterprise has to:
- Outline Standards: perceive your online business wants and goals. Determine the precise duties and outcomes you plan to realize with the mannequin. Clearly outline what profitable mannequin efficiency appears to be like like for every activity, and set up parameters for acceptable outcomes and behaviors to make sure the mannequin aligns along with your expectations.
- Slender Down Your Mannequin Choices: Filter fashions based mostly on their perform, complexity, and suitability on your particular duties. Take into account fashions which have established monitor information for duties much like yours, similar to coding-specific fashions for software program growth.
- Collect/Curate Information: Gather information that simulates the standard interactions your mannequin will deal with. If needed, generate artificial information to make sure it aligns along with your analysis standards.
- Run Evaluations: Check every shortlisted mannequin towards your outlined standards. Experiment with totally different mannequin and immediate mixtures to acquire probably the most complete outcomes.
And that’s simply scratching this floor. There’s fairly a bit mor that goes into making the precise selection
The Analysis Dilemma
Evaluating new fashions is not any easy activity. It requires a deep understanding of the mannequin’s structure, the information it was educated on, and its efficiency on related benchmarks. However even with this data, there’s no assure {that a} mannequin will seamlessly combine into your current infrastructure or meet your online business wants.
The method is time-consuming and resource-intensive, and if not approached systematically, can simply result in useless ends. For instance:
- What if none of those fashions meet my success standards?
- What if the immediate I perfected for mannequin A seems to be ineffective for mannequin B? (Not each immediate is profitable for each LLM)
- Do I now must fine-tune my very own mannequin to get the outcomes I would like?
At this level, it’d be simple to grasp if an organization regrets having gone down this path in any respect.
It’s Not Concerning the Mannequin; It’s About Your Information
Whereas it’s simple to be dazzled by the most recent and newest, the most recent mannequin isn’t at all times the simplest resolution on your distinctive use case.
Backside line: customizability is extra necessary than uncooked functionality. That means, simply because mannequin benchmarks (which aren’t based mostly in your group’s information) present that it performs higher than its predecessor, it doesn’t imply it’s going to truly carry out properly for you.
Novelty doesn’t assure compatibility along with your information, nor does it imply it’s going to scale and really drive significant enterprise outcomes.
That’s why it’s completely vital to observe the steps outlined above earlier than making any vital funding. You might want to perceive what the target is first and go from there. Failing to put the groundwork may render the mannequin analysis part meaningless.
In the long run, the outcomes on your app and your prospects are what actually issues; work backwards from there. Curate the perfect information particular to your activity and measure success towards that alone. Generic benchmarks received’t provide the solutions it is advisable make the precise selection.
Concerning the writer: Luis Ceze is the CEO and co-founder OctoAI and a pc professor on the College of Washington.
Associated Objects:
Coming to Grips with Immediate Lock-In
The Way forward for AI Is Hybrid
Birds Aren’t Actual. And Neither Is MLOps
[ad_2]