[ad_1]
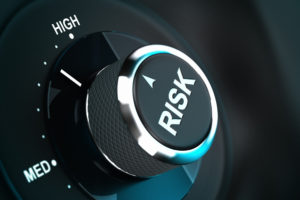
(Olivier Le Moal/Shutterstock)
The Cloud Safety Alliance (CSA), a corporation devoted to defining and elevating consciousness of finest practices to assist guarantee a safe cloud computing surroundings, launched a brand new paper that gives pointers for the accountable growth, deployment, and use of AI fashions
The report, titled “Synthetic Intelligence (AI) Mannequin Threat Administration Framework,” showcases the crucial position of mannequin danger administration (MRM) in making certain moral, environment friendly, and accountable AI use.
“Whereas the growing reliance on AI/ML fashions holds the promise of unlocking the huge potential for innovation and effectivity features, it concurrently introduces inherent dangers, significantly these related to the fashions themselves, which if left unchecked can result in important monetary losses, regulatory sanctions, and reputational harm. Mitigating these dangers necessitates a proactive method similar to that outlined on this paper,” stated Vani Mittal, a member of the AI Know-how & Threat Working Group and a lead writer of the paper.
The most typical AI mannequin dangers embrace information high quality points, implementation and operation errors, and intrinsic dangers similar to information biases, factual inaccuracies, and hallucinations.
A complete AI danger administration framework can handle these challenges with elevated transparency, accountability, and decision-making. The framework can even allow focused danger mitigation, steady monitoring, and sturdy mannequin validation to make sure fashions stay efficient and reliable.
The paper current 4 core pillars of an efficient mannequin danger administration (MRM) technique: Mannequin Playing cards, Information Sheets, Threat Playing cards, and Situation Planning. It additionally highlights how these parts work collectively to determine and mitigate dangers and enhance mannequin growth by way of a steady suggestions loop.
Mannequin Playing cards element the meant goal, coaching information composition, identified limitations, and different metrics to assist perceive the strengths and weaknesses of the mannequin. It serves as a basis for the chance administration framework.
The Information Sheets element offers an in depth technical description of machine studying (ML) fashions together with key insights into the operational traits, mannequin structure, and growth course of. This pillar serves as a technical roadmap for the mannequin’s building and operation, enabling danger administration professionals to successfully assess, handle, and govern dangers related to the ML fashions.
After the potential points have been recognized, Threat Playing cards are used to delve deeper into the problems. Every Threat Card describes a selected danger, potential affect, and mitigation methods. Threat Playing cards permit for a dynamic and structured method to managing the quickly evolving panorama of mannequin danger.
The final element, Situation Planning, is used as a proactive method to inspecting hypothetical conditions by which an AI mannequin is perhaps misused or experiences a malfunction. This enables danger administration professionals to determine potential points earlier than they turn out to be actuality.
The true effectiveness of the chance administration framework comes from the deep integration of the 4 parts to kind a holistic technique. For instance, the data from the Mannequin Playing cards helps create Information Sheets that feed important insights to create Threat Playing cards to handle every danger individually. The continued suggestions loop of the MRM is essential to refining danger assessments and creating danger mitigation methods.
As AI and ML advance, mannequin danger administration (MRM) practices should preserve tempo. Based on CSA, the long run updates to the paper will give attention to refining the framework by creating standardized paperwork for the 4 pillars, integrating MLOps and automation, navigating regulatory challenges, and enhancing AI explainability.
Associated Objects
Why the Present Strategy for AI Is Excessively Harmful
NIST Places AI Threat Administration on the Map with New Framework
Regs Wanted for Excessive-Threat AI, ACM Says–‘It’s the Wild West’
[ad_2]