[ad_1]
Information at a Look
- Meta broadcasts Chameleon, a complicated multimodal giant language mannequin (LLM).
- Chameleon makes use of an early-fusion token-based mixed-modal structure.
- The mannequin processes and generates textual content and pictures inside a unified token house.
- It outperforms different fashions in duties like picture captioning and visible query answering (VQA).
- Meta goals to proceed enhancing Chameleon and exploring further modalities.
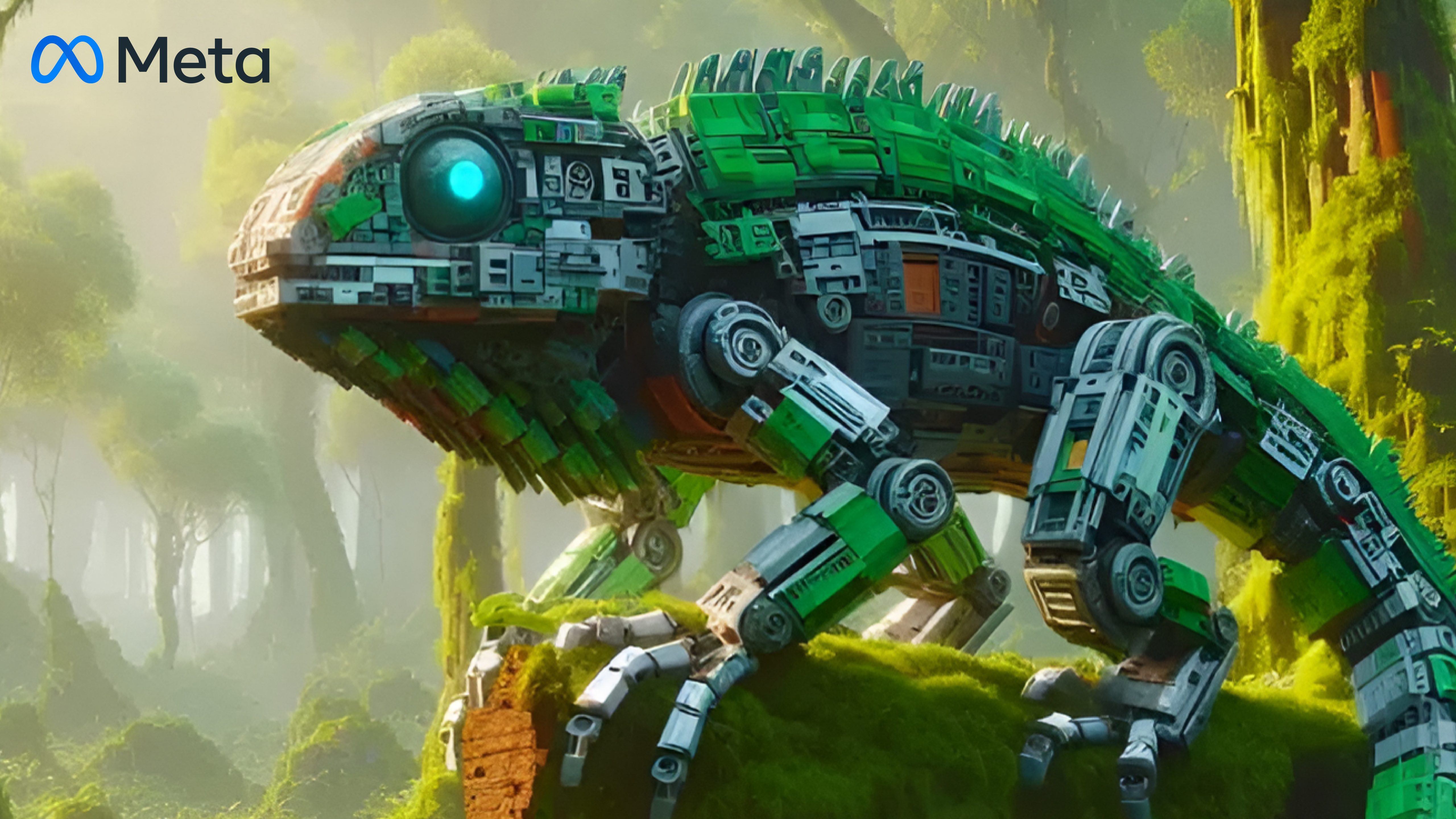
Meta is making strides in synthetic intelligence (AI) with a brand new multimodal LLM named Chameleon. This mannequin, primarily based on early-fusion structure, guarantees to combine various kinds of info higher than its predecessors. With this transfer, Meta is positioning itself as a powerful contender within the AI world.
Additionally Learn: Ray-Ban Meta Sensible Glasses Get a Multimodal AI Improve
Understanding Chameleon’s Structure
Chameleon employs an early-fusion token-based mixed-modal structure, setting it other than conventional fashions. Not like the late-fusion method, the place separate fashions course of totally different modalities earlier than combining them, Chameleon integrates textual content, photos, and different inputs from the beginning. This unified token house permits Chameleon to motive over and generate interleaved sequences of textual content and pictures seamlessly.
Meta’s researchers spotlight the mannequin’s progressive structure. By encoding photos into discrete tokens much like phrases in a language mannequin, Chameleon creates a blended vocabulary that features textual content, code, and picture tokens. This design permits the mannequin to use the identical transformer structure to sequences containing each picture and textual content tokens. It enhances the fashions’s means to carry out duties that require a simultaneous understanding of a number of modalities.
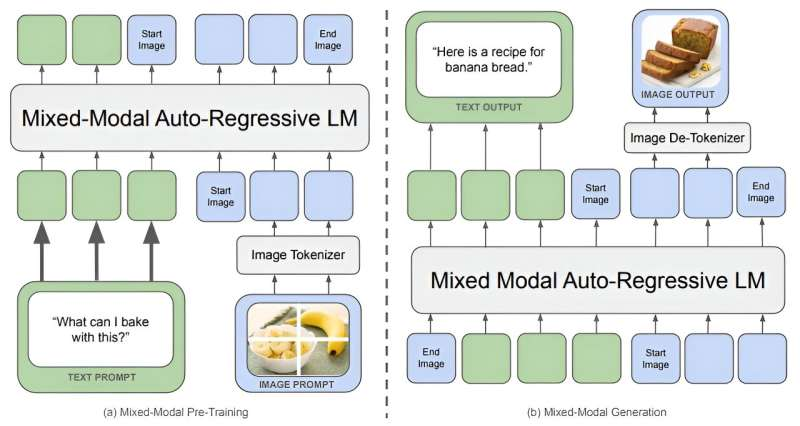
Coaching Improvements and Methods
Coaching a mannequin like Chameleon presents vital challenges. To deal with these, Meta’s workforce launched a number of architectural enhancements and coaching strategies. They developed a novel picture tokenizer and employed strategies corresponding to QK-Norm, dropout, and z-loss regularization to make sure steady and environment friendly coaching. The researchers additionally curated a high-quality dataset of 4.4 trillion tokens, together with textual content, image-text pairs, and interleaved sequences.
Chameleon’s coaching occurred in two levels, with variations of the mannequin boasting 7 billion and 34 billion parameters. The coaching course of spanned over 5 million hours on Nvidia A100 80GB GPUs. These efforts have resulted in a mannequin able to performing numerous text-only and multimodal duties with spectacular effectivity and accuracy.
Additionally Learn: Meta Llama 3: Redefining Giant Language Mannequin Requirements
Efficiency Throughout Duties
Chameleon’s efficiency in vision-language duties is notable. It surpasses fashions like Flamingo-80B and IDEFICS-80B in picture captioning and VQA benchmarks. Moreover, it competes properly in pure textual content duties, reaching efficiency ranges akin to state-of-the-art language fashions. The mannequin’s means to generate mixed-modal responses with interleaved textual content and pictures units it other than its opponents.
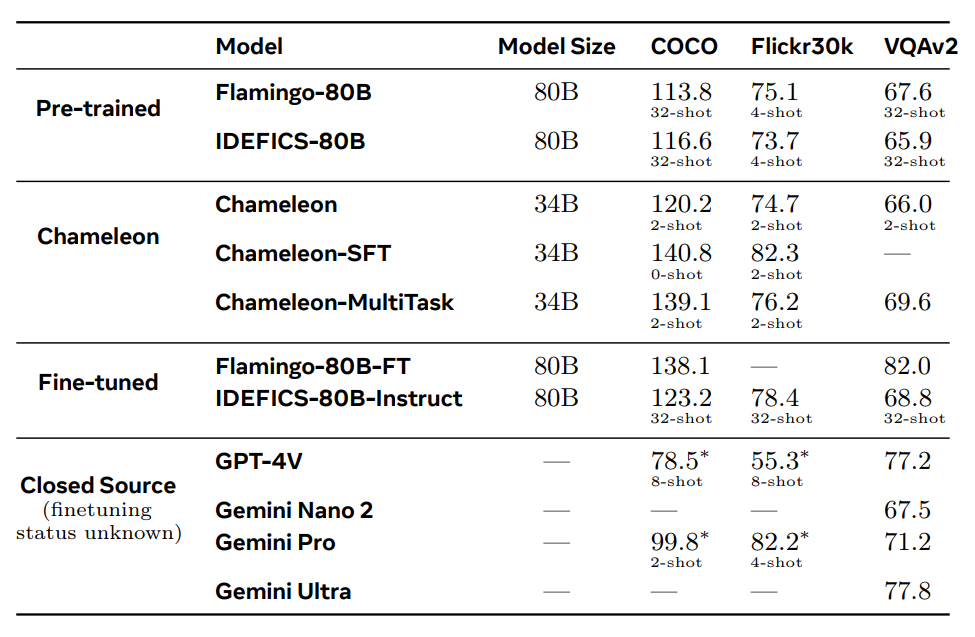
Meta’s researchers report that Chameleon achieves these outcomes with fewer in-context coaching examples and smaller mannequin sizes, highlighting its effectivity. The mannequin’s versatility and functionality to deal with mixed-modal reasoning make it a precious instrument for numerous AI functions, from enhanced digital assistants to classy content-generation instruments.
Future Prospects and Implications
Meta sees Chameleon as a big step in direction of unified multimodal AI. Going ahead, the corporate plans to discover the mixing of further modalities, corresponding to audio, to additional improve its capabilities. This might open doorways to a variety of recent functions that require complete multimodal understanding.
Chameleon’s early-fusion structure can also be fairly promising, particularly in fields corresponding to robotics. Researchers might doubtlessly develop extra superior and responsive AI-driven robots by integrating this know-how into their management methods. The mannequin’s means to deal with multimodal inputs might additionally result in extra subtle interactions and functions.
Our Say
Meta’s introduction of Chameleon marks an thrilling growth within the multimodal LLM panorama. Its early-fusion structure and spectacular efficiency throughout numerous duties spotlight its potential to revolutionize multimodal AI functions. As Meta continues to boost and increase Chameleon’s capabilities, it might set a brand new normal in AI fashions for integrating and processing numerous varieties of info. The longer term appears promising for Chameleon, and we anticipate seeing its impression on numerous industries and functions.
Comply with us on Google Information to remain up to date with the most recent improvements on the planet of AI, Information Science, & GenAI.
[ad_2]