[ad_1]
Within the quickly evolving panorama of Generative AI (GenAI), knowledge scientists and AI builders are continually in search of highly effective instruments to create modern purposes utilizing Giant Language Fashions (LLMs). DataRobot has launched a set of superior LLM analysis, testing, and evaluation metrics of their Playground, providing distinctive capabilities that set it aside from different platforms.
These metrics, together with faithfulness, correctness, citations, Rouge-1, price, and latency, present a complete and standardized strategy to validating the standard and efficiency of GenAI purposes. By leveraging these metrics, prospects and AI builders can develop dependable, environment friendly, and high-value GenAI options with elevated confidence, accelerating their time-to-market and gaining a aggressive edge. On this weblog submit, we are going to take a deep dive into these metrics and discover how they might help you unlock the complete potential of LLMs throughout the DataRobot platform.
Exploring Complete Analysis Metrics
DataRobot’s Playground presents a complete set of analysis metrics that enable customers to benchmark, examine efficiency, and rank their Retrieval-Augmented Technology (RAG) experiments. These metrics embrace:
- Faithfulness: This metric evaluates how precisely the responses generated by the LLM replicate the info sourced from the vector databases, guaranteeing the reliability of the data.
- Correctness: By evaluating the generated responses with the bottom reality, the correctness metric assesses the accuracy of the LLM’s outputs. That is notably invaluable for purposes the place precision is essential, equivalent to in healthcare, finance, or authorized domains, enabling prospects to belief the data supplied by the GenAI utility.
- Citations: This metric tracks the paperwork retrieved by the LLM when prompting the vector database, offering insights into the sources used to generate the responses. It helps customers be certain that their utility is leveraging probably the most acceptable sources, enhancing the relevance and credibility of the generated content material.The Playground’s guard fashions can help in verifying the standard and relevance of the citations utilized by the LLMs.
- Rouge-1: The Rouge-1 metric calculates the overlap of unigram (every phrase) between the generated response and the paperwork retrieved from the vector databases, permitting customers to guage the relevance of the generated content material.
- Price and Latency: We additionally present metrics to trace the price and latency related to operating the LLM, enabling customers to optimize their experiments for effectivity and cost-effectiveness. These metrics assist organizations discover the correct stability between efficiency and finances constraints, guaranteeing the feasibility of deploying GenAI purposes at scale.
- Guard fashions: Our platform permits customers to use guard fashions from the DataRobot Registry or customized fashions to evaluate LLM responses. Fashions like toxicity and PII detectors may be added to the playground to guage every LLM output. This permits straightforward testing of guard fashions on LLM responses earlier than deploying to manufacturing.
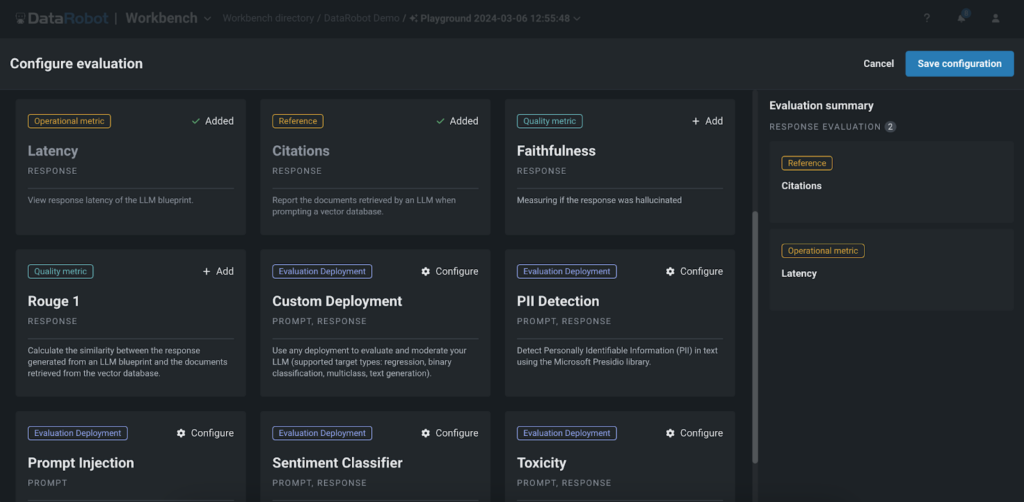
Environment friendly Experimentation
DataRobot’s Playground empowers prospects and AI builders to experiment freely with completely different LLMs, chunking methods, embedding strategies, and prompting strategies. The evaluation metrics play a vital position in serving to customers effectively navigate this experimentation course of. By offering a standardized set of analysis metrics, DataRobot allows customers to simply examine the efficiency of various LLM configurations and experiments. This enables prospects and AI builders to make data-driven selections when choosing the right strategy for his or her particular use case, saving time and assets within the course of.
For instance, by experimenting with completely different chunking methods or embedding strategies, customers have been capable of considerably enhance the accuracy and relevance of their GenAI purposes in real-world eventualities. This stage of experimentation is essential for creating high-performing GenAI options tailor-made to particular business necessities.
Optimization and Consumer Suggestions
The evaluation metrics in Playground act as a invaluable device for evaluating the efficiency of GenAI purposes. By analyzing metrics equivalent to Rouge-1 or citations, prospects and AI builders can establish areas the place their fashions may be improved, equivalent to enhancing the relevance of generated responses or guaranteeing that the applying is leveraging probably the most acceptable sources from the vector databases. These metrics present a quantitative strategy to assessing the standard of the generated responses.
Along with the evaluation metrics, DataRobot’s Playground permits customers to offer direct suggestions on the generated responses by thumbs up/down scores. This consumer suggestions is the first methodology for making a fine-tuning dataset. Customers can evaluation the responses generated by the LLM and vote on their high quality and relevance. The up-voted responses are then used to create a dataset for fine-tuning the GenAI utility, enabling it to be taught from the consumer’s preferences and generate extra correct and related responses sooner or later. Which means that customers can gather as a lot suggestions as wanted to create a complete fine-tuning dataset that displays real-world consumer preferences and necessities.
By combining the evaluation metrics and consumer suggestions, prospects and AI builders could make data-driven selections to optimize their GenAI purposes. They’ll use the metrics to establish high-performing responses and embrace them within the fine-tuning dataset, guaranteeing that the mannequin learns from the perfect examples. This iterative technique of analysis, suggestions, and fine-tuning allows organizations to repeatedly enhance their GenAI purposes and ship high-quality, user-centric experiences.
Artificial Knowledge Technology for Speedy Analysis
One of many standout options of DataRobot’s Playground is the artificial knowledge era for prompt-and-answer analysis. This characteristic permits customers to shortly and effortlessly create question-and-answer pairs based mostly on the consumer’s vector database, enabling them to totally consider the efficiency of their RAG experiments with out the necessity for handbook knowledge creation.
Artificial knowledge era presents a number of key advantages:
- Time-saving: Creating massive datasets manually may be time-consuming. DataRobot’s artificial knowledge era automates this course of, saving invaluable time and assets, and permitting prospects and AI builders to quickly prototype and take a look at their GenAI purposes.
- Scalability: With the power to generate hundreds of question-and-answer pairs, customers can totally take a look at their RAG experiments and guarantee robustness throughout a variety of eventualities. This complete testing strategy helps prospects and AI builders ship high-quality purposes that meet the wants and expectations of their end-users.
- High quality evaluation: By evaluating the generated responses with the artificial knowledge, customers can simply consider the standard and accuracy of their GenAI utility. This accelerates the time-to-value for his or her GenAI purposes, enabling organizations to convey their modern options to market extra shortly and acquire a aggressive edge of their respective industries.
It’s essential to contemplate that whereas artificial knowledge offers a fast and environment friendly strategy to consider GenAI purposes, it could not at all times seize the complete complexity and nuances of real-world knowledge. Subsequently, it’s essential to make use of artificial knowledge along side actual consumer suggestions and different analysis strategies to make sure the robustness and effectiveness of the GenAI utility.
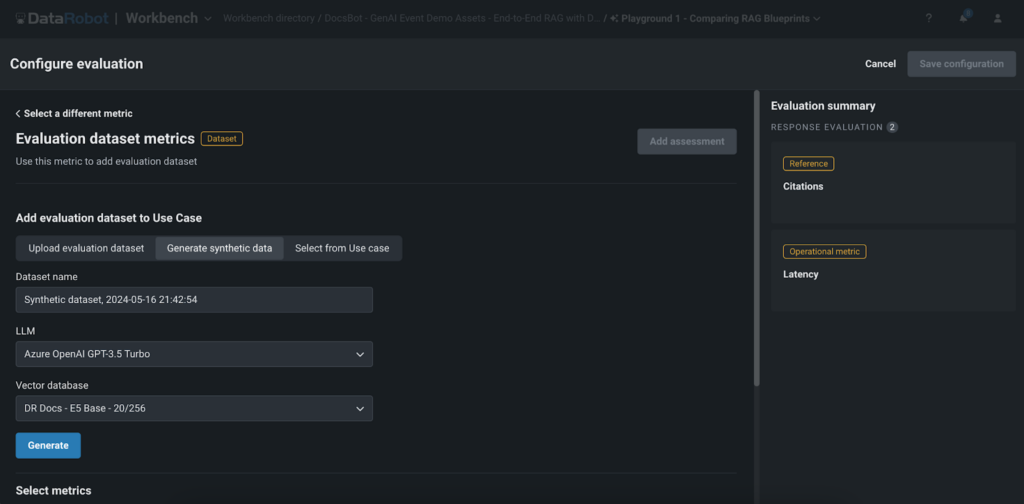
Conclusion
DataRobot’s superior LLM analysis, testing, and evaluation metrics in Playground present prospects and AI builders with a strong toolset to create high-quality, dependable, and environment friendly GenAI purposes. By providing complete analysis metrics, environment friendly experimentation and optimization capabilities, consumer suggestions integration, and artificial knowledge era for fast analysis, DataRobot empowers customers to unlock the complete potential of LLMs and drive significant outcomes.
With elevated confidence in mannequin efficiency, accelerated time-to-value, and the power to fine-tune their purposes, prospects and AI builders can concentrate on delivering modern options that remedy real-world issues and create worth for his or her end-users. DataRobot’s Playground, with its superior evaluation metrics and distinctive options, is a game-changer within the GenAI panorama, enabling organizations to push the boundaries of what’s potential with Giant Language Fashions.
Don’t miss out on the chance to optimize your initiatives with probably the most superior LLM testing and analysis platform out there. Go to DataRobot’s Playground now and start your journey in direction of constructing superior GenAI purposes that really stand out within the aggressive AI panorama.
Concerning the writer
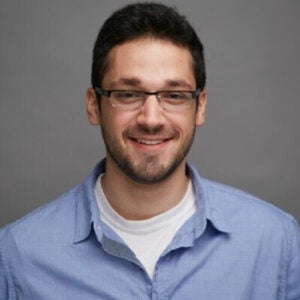
Nathaniel Daly is a Senior Product Supervisor at DataRobot specializing in AutoML and time sequence merchandise. He’s centered on bringing advances in knowledge science to customers such that they will leverage this worth to unravel actual world enterprise issues. He holds a level in Arithmetic from College of California, Berkeley.
[ad_2]