Managing and Understanding Participant Suggestions at Scale
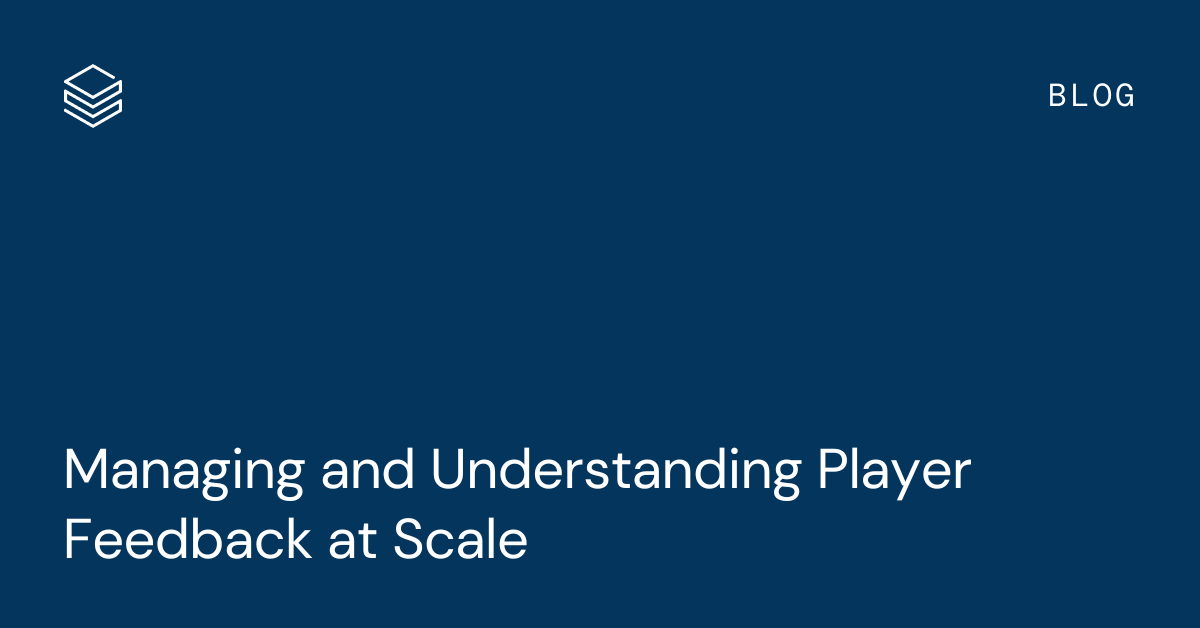
Whether or not you’re engaged on a dwell title, pre/submit manufacturing, ongoing upkeep, future releases, one other model of a recreation, or a model new title for the market, you are all the time searching for suggestions from the group. There isn’t any scarcity of it on the market, however it may be overwhelming and arduous to sift by way of. For video games shipped on PC and bought by way of Valve’s Steam Retailer, an excellent supply of participant suggestions in your title could be present in Steam’s recreation opinions. We’ve constructed a brand new answer accelerator for Participant Assessment Evaluation that mixes pure languages and machine studying strategies to assist recreation builders perceive their gamers higher and reply by way of their recreation design, backend operations, LiveOperations, Advertising and marketing and, actually, by way of all traces of enterprise.
With Steam’s recreation opinions, you’ve got the chance to see:
- Uncooked suggestions: The participant’s unprompted phrases. What they felt most keen about: constructive or damaging
- Suggestions over time: For gamers as a complete, and even particular person gamers
- Suggestions as a relation to time performed: When are folks most constructive? In the event that they solely performed for 4 hours? In the event that they hit 100%?
- Suggestions for different titles: What are the issues that folks harp on essentially the most when speaking about an aRPG, or an RTS? Does it differ relying on A, AA, AAA+?
Say you have gathered this suggestions, you have acquired it in your knowledge platform, what’s subsequent? How does one make sense of all of it? Studying by way of tons of, or hundreds of plain textual content opinions (unstructured knowledge) to reliably discover patterns and or insights could be daunting.
That is the place the facility of pure language processing is available in. With this machine studying (ML) answer you’ll be able to extract the important thing phrases and their related constructive, impartial, or damaging sentiment. Utilizing ML, you’ll be able to mitigate biases and see what the information is de facto making an attempt to inform you. This perception can occur at an aggregated or participant particular stage. When analyzing your individual title, you should have entry to your Participant ID and be capable of align that with Steam’s Recreation ID. With this, you’ll be able to increase your player360 datasets with the sentiment expressed on Steam enabling you to proactively take motion to enhance engagement, retention and income metrics.
Think about a excessive worth participant has simply dropped an extremely damaging evaluation. The earlier that you just notice that connection, the quicker you’ll be able to take motion to mitigate what is going on on, interact with the participant (and broader group) immediately and enhance your possibilities to retain them. Such a evaluation is very vital for dwell service titles, shipped in cycles of fixed iteration.
The perception derived is helpful throughout the board:
- Backend Operations: What elements of the backend are driving frustration? Is it lag, server stability, matchmaking time? Superior: How might we allocate our backend sources to enhance the efficiency for prime worth gamers experiencing these points?
- Group and Help: Determine sources of friction for gamers. What’s driving them mad? Degree up: Construct out responses to the highest points gamers are experiencing in order that Group Managers and Help can reply in a significant method and, ideally, allay considerations primarily based on deliberate enhancements.
- Recreation Design: What do folks really feel is weak, or overpowered (OP)? What recreation modes are they having fun with essentially the most? Which modes would they like extra of, however perhaps aren’t hitting the mark at the moment? Why aren’t they hitting the mark? Superior: Cross reference recommended enhancements in opposition to inside participant segmentation, play time and different cross-org knowledge factors.
- Advertising and marketing: Why are folks loving your recreation? What’s getting them excited, once you have a look at the constructive opinions, what are the traits, why are they participating? Take this perception and align your advert creatives, advert/e-mail campaigns and re-engagement strategies to what’s most fun in your gamers. Superior: Combine participant segmentation throughout income, play model, and different views to create section+pleasure centered outreach that feels personalised to the participant.
- LiveOperations: How are your LiveOperations occasions being acquired? Which of them are folks most enthusiastic about, or dissatisfied in? You will see the web impact of this by way of income transactions throughout an occasion, however you will not get the feels there. Right here you may perceive the explanation for these income outcomes. Superior: Explicitly be a part of occasion centered suggestions with income outcomes in your occasions and operational challenges. Your occasion might need been nice however you had main server points impacting one geographical section who turned the vocal minority in your opinions. Solely by becoming a member of these disparate insights would you see that the opinions are steering you within the mistaken route (from an occasion perspective) and it is actually a backend operations problem to handle.
Now that we perceive the why, the how and the affect, let’s get to enjoyable stuff!
Within the beneath sections we’ll stroll by way of methods to take varied opinions from Steam and course of then curate unstructured textual content into actionable knowledge.
Notice: Although we solely cowl Steam the identical sample could be utilized to many different sources of knowledge.
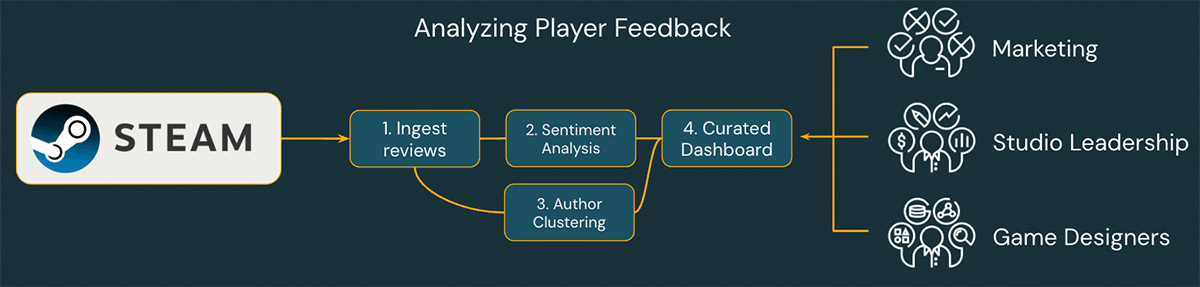
1. Information Ingestion and Social Media APIs
Within the knowledge ingestion section of the sentiment evaluation answer, we make the most of the Steam API to assemble gaming opinions. This uncooked knowledge is cleaned to take away any irrelevant or corrupt knowledge, and filtered to incorporate solely these opinions written in English. This cleaned and filtered knowledge is saved within the bronze layer of our knowledge pipeline, serving because the foundational dataset for subsequent evaluation phases.
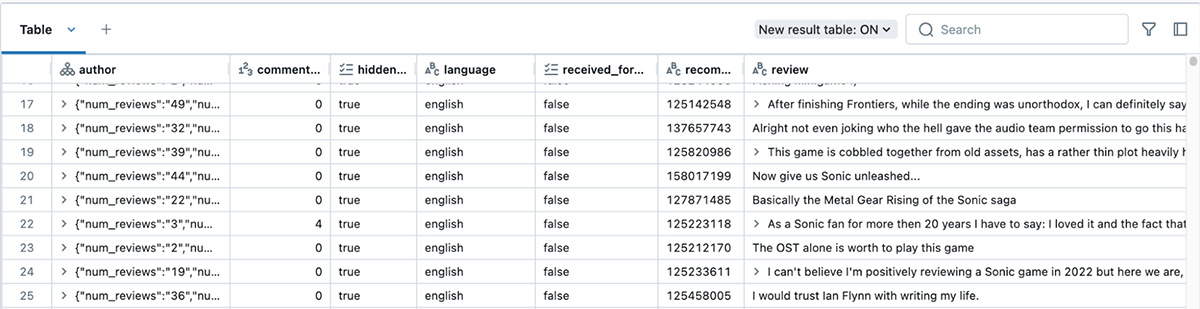
2. Sentiment Evaluation Pipeline
On this part, we create an information processing pipeline utilizing Spark NLP. It begins by structuring and cleansing the textual content, then identifies sentences and breaks them into particular person phrases, guaranteeing uniformity in illustration. After standardizing the phrases and eradicating frequent however non-informative phrases, it enriches the textual content by embedding phrases right into a numerical vector house, facilitating deeper linguistic evaluation. Moreover, it leverages a pre-trained mannequin from John Snow Labs to routinely detect constructive, damaging and impartial facets concerning the recreation from person opinions. As a substitute of labeling the complete evaluation as damaging or constructive, this mannequin helps determine the sentiment of actual phrases associated within the evaluation.
3. Writer Primarily based Clustering
Shifting to the following part of our sentiment evaluation answer, we make use of k-means clustering to section the authors of the gaming opinions primarily based on their metadata. This clustering is executed utilizing PySpark’s MLlib, which effectively handles massive datasets by distributing the computation throughout a number of nodes. This segmentation provides a layer of granularity to our dashboard, enabling deeper insights into totally different person demographics and behaviors.
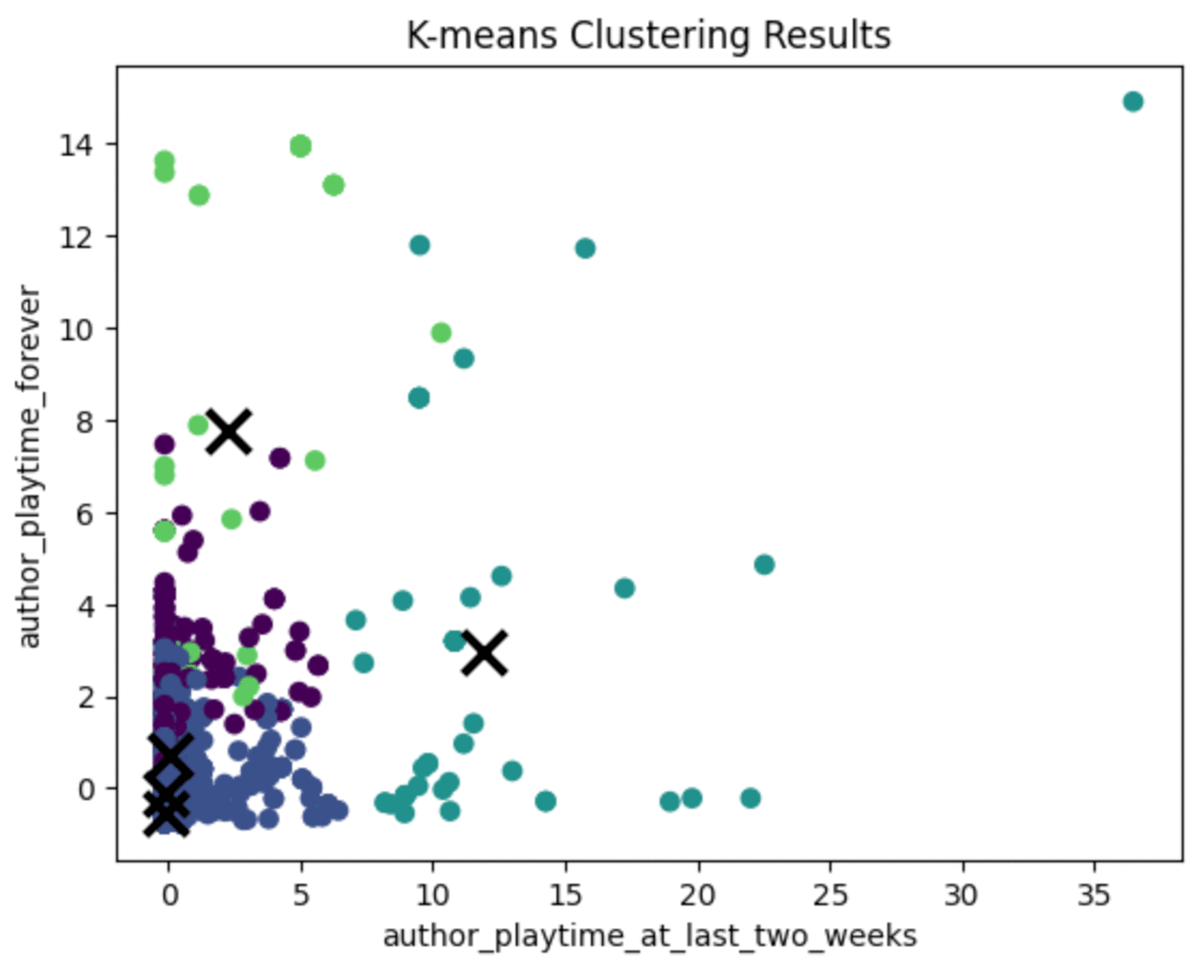
4. Analyzing the Outcomes
Now that you’ve got your labeled knowledge you can also make use of all of it. A product supervisor may have a look at this dataset and see excessive negativity associated to a selected recreation function and regulate their pipeline to handle that extra shortly. Somebody in operations may have a look at the concentrations of places for folks complaining about server drop outs throughout totally different geographies to determine potential multiplayer server orchestration points throughout markets. A LiveOps content material creator may discover extra positivity on BFGs and make investments extra time constructing skins for these merchandise.
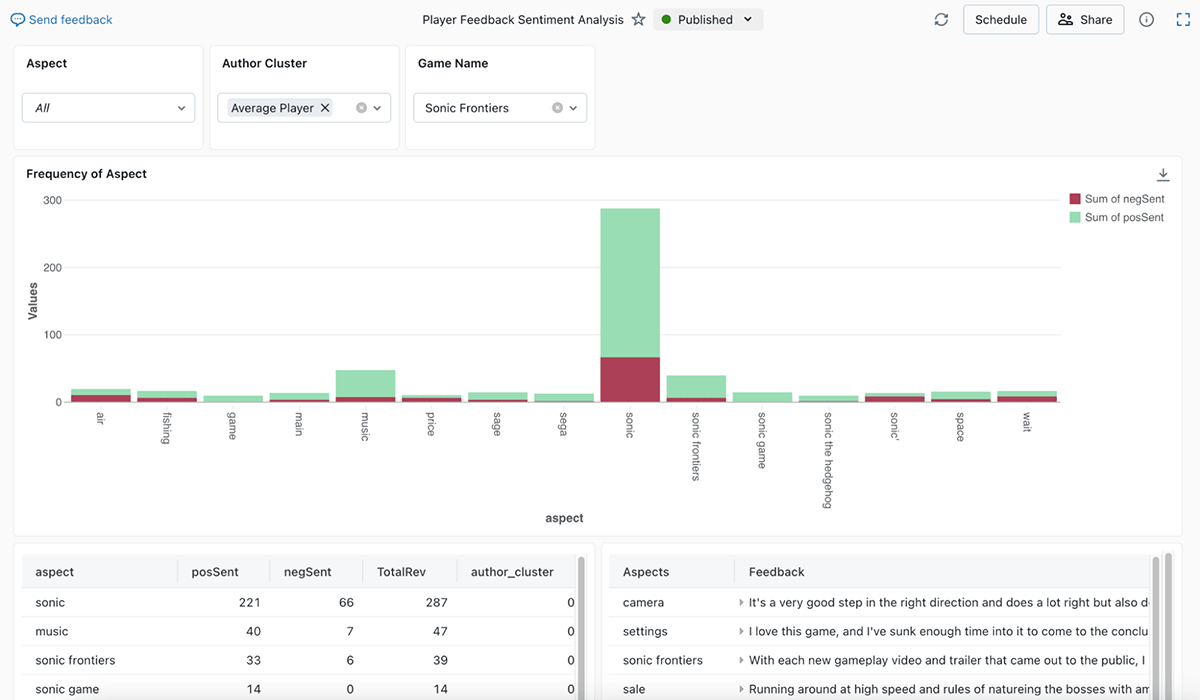
5. Take Benefit of Your New Dataset
You now have a dataset that provides you perception into what your gamers are saying at scale. This may very well be used to assist personalize the expertise of your gamers and enhance retention. By taking this as an enter, connecting it to your inside datasets on engagement and income you’ll be able to inform motion by group managers, buyer assist, advertising and provide suggestions. Buying gamers is pricey, discovering the gamers you need to maintain is difficult, this perception supplies a chance to interact together with your group and construct a deeper relationship with them and, by doing so, enhance your participant retention.
Conclusion
This answer accelerator for Participant Assessment Evaluation combines pure languages and machine studying strategies to assist recreation builders perceive their gamers higher and reply by way of their recreation design, backend operations, LiveOperations, Advertising and marketing and, actually, by way of all traces of enterprise. A recreation firm, in pre-production, trying to construct one thing new may analyze comparable video games to seek out scorching buttons (constructive and damaging) for his or her goal gamers. A studio throughout beta could use it to shortly reply to suggestions throughout all gamers, or submit launch to constantly enhance the title over time and maximize engagement.
This answer accelerator is targeted on the evaluation of Steam opinions, however that is only one knowledge supply. This strategy can be utilized to research opinions from different websites, boards, assist tickets, surveys, certainly any plain textual content suggestions you’ve got entry to. So long as you’ll be able to accumulate, and ingest it into this workflow/system, it may be used.
Suggestions is a present. We’re excited to assist these voices be heard, develop participant engagement and help as you additional the enjoyable.
Prepared for extra recreation knowledge + AI use circumstances?
Obtain our Final Information to Recreation Information and AI. This complete eBook supplies an in-depth exploration of the important thing matters surrounding recreation knowledge and AI, from the enterprise worth it supplies to the core use circumstances for implementation. Whether or not you are a seasoned knowledge veteran or simply beginning out, our information will equip you with the information it’s worthwhile to take your recreation growth to the following stage.